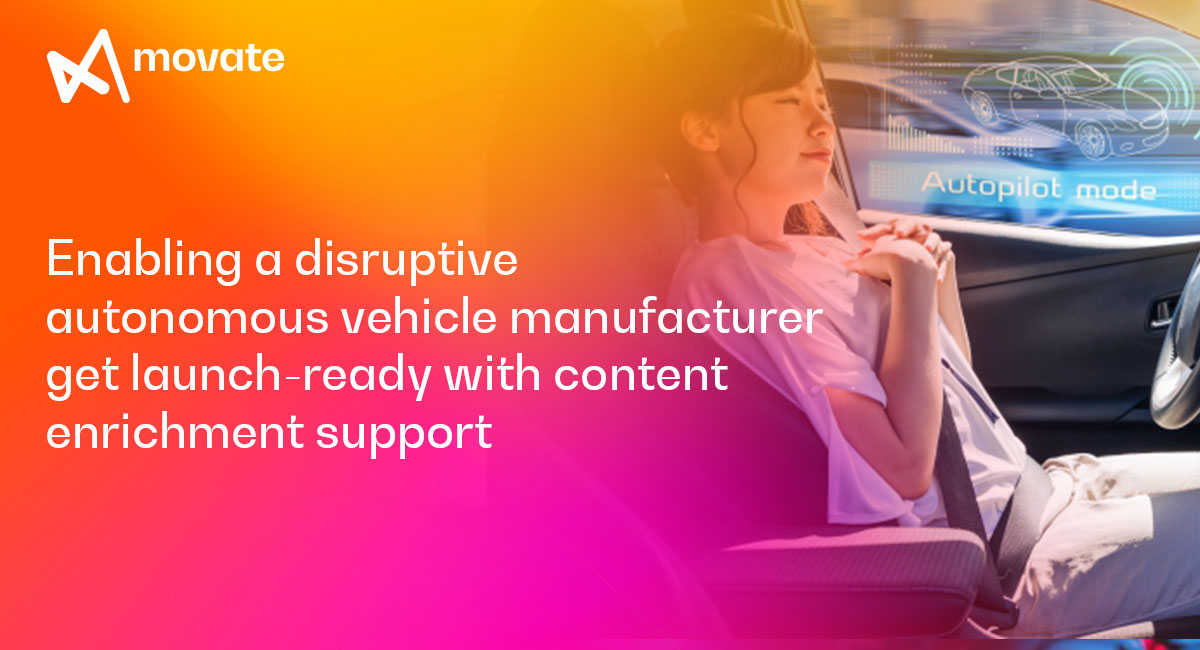
About the client
The client is a US-based company and one among the many players in the autonomous vehicle markets. They develop and manufacture self-driving vehicles aimed at urban mobility giving passengers a unique driving experience.
Client challenge
They wanted to get market ready products at optimal costs while enabling superior, technology-laced driving and CX for passengers. They needed help with content labeling and annotation of 3D object and LiDAR data, and were looking at a partner who could provide them with rapid deployment.
Movate solution
Movate arrived the following scope of work:
- Co-innovating with client engineering team to improve platform capability
- Enabling client in pre-launch phase for accelerated market readiness
- Scope included end-to-end data annotation/ labelling support for identified US cities (San Francisco, Los Angeles, Seattle) and Europe street data
Creating knowledge-base datasets
Movate began by co-innovating with the client’s engineering team to advance learning and technology application to fast forward the platforms’ capabilities. The team created a comprehensive knowledgebase that comprised of real-world data taken from the respective cities which is ingested into the client’s labeling and annotation platform. The data is used to provide detailed attributes to describe the objects.
The team uses Cuboid annotation – a data labeling technique where sampled 2D data is used to create 3D representational models. This process helps autonomous systems clearly differentiate between different objects in a real-world scene by taking help of the annotations and labels used to describe the data. Using cuboids, the AI program can also map out basic vectors like length, width, height, depth etc to distinguish between objects. This data is got through LiDAR (Light Detection and Ranging). The annotation takes place within a 3D environment but is backed up by real-world data to ensure consistency and accuracy of all objects. By doing this process, we are creating knowledge-base datasets that the AI/ML program can then use on the go. The data that is got (usually ranges in TBs – terabytes) included the following:
- Object detection
- Face detection
- Road elements detection
- Vehicle detection
- Humans & animal detections
As an additional step, the team manually verifies the quality effectiveness of the tagged data to ensure there’s a proper QC on its integrity.
We also helped the client accelerate their go-to-market phase by continuously testing and updating their different modules within their platform to become more intuitive, efficient and robust and help the client overcome all known obstacles and can be ready to deploy. This is an ongoing process. We also created an extensive knowledge management system to take care of the annotations and labeling generated through an easy, lookup interface for faster access.
Business Benefits
- $75K in cost savings annually with potential of further incremental savings
- 20% improvement in productivity
- 99.8% quality assurance of generated image and object labelling
- 25% improvement in rider experience among beta users