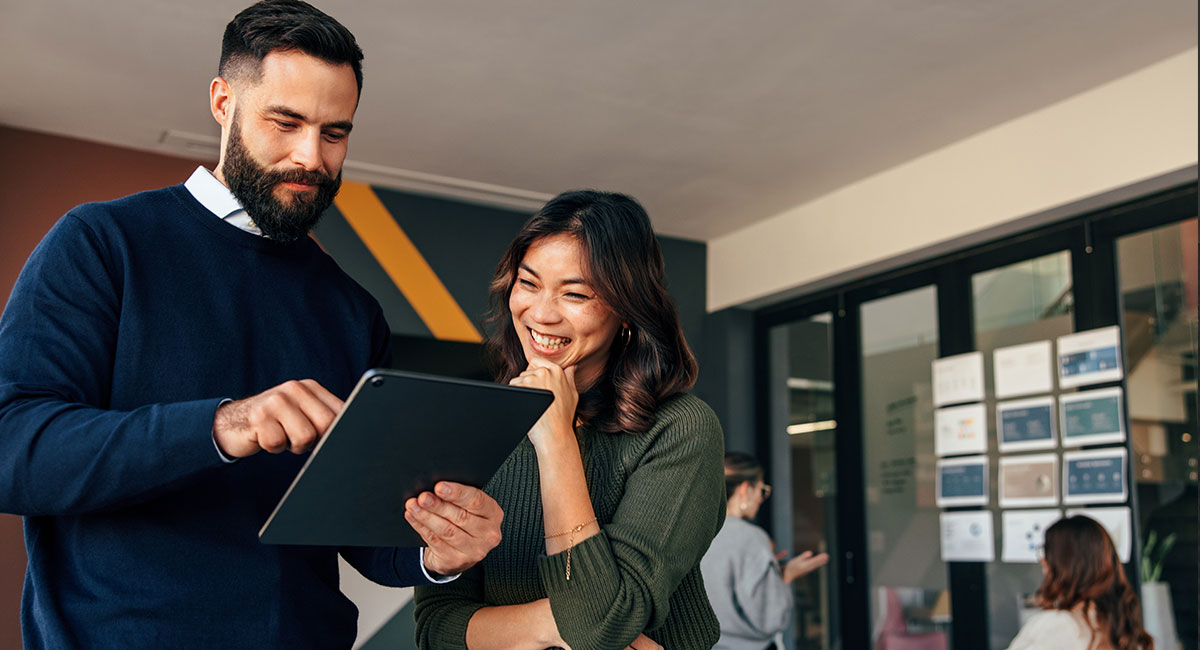
01 Introduction
The demand and dominance of technology have never reached heights like before. Today, everything we do as consumers or within a business has an imprint of some tech framework. And digitalization has exacerbated the need to thrive or perish. With an ever-evolving technological core, enterprises are constantly facing the balance of power between upgrading their technical infrastructure and viz powering memorable experiences for customers. This POV looks at the five most promising, accepted technology frameworks that will transform the way enterprises work in the future.
02 Trend #1:
Generative AI
The significance of AI (Artificial Intelligence) has caught up, and the world has seen its transformative eects in action. Generative AI is the new kid on the block that uses AI, large models, and datasets, through which it is trained to handle and accept data and process them more like humans do. By training the application this way, generative AI can converse with near-human accuracy and engage in meaningful conversations.
Backed by training and modeling and access to a heterogenous set of data and models, AI can help enterprises in many functions, such as customer support, testing, application development, and smart automation that accelerates many functions in an enterprise space.
AI specifically trained to write application codes is called TuringBots (an ode to Alan Turing – the father of artificial intelligence.) A Forrester report says, “TuringBots will write 10% of worldwide codes and tests by leveraging AI & ML and existing applications codes.” This will eventually transform an application developer’s profile from coding to managing and overseeing development because most of the hard work will be automated with checks and balances in place.
2.1 Generative AI has the power to transform content marketing
Riding on its popularity, generative AI is all set to transform marketing and content creation by providing stimulating forms of interactive and immersive content using a mesh of multimedia formats that span audio, video, images, and text and tapping into a large conversation data model to produce human-like content. There are many examples to showcase, like (Chat GPT, Google BERT, and DALL-E).
2.2 Explainable AI (XAI) to address risks and bring transparency to enterprise AI
While AI and its applications have the potential to augment humans in their work, the lack of a clear understanding of how these applications perform creates a sense of distrust when these models are used in real-world productions. Explainable AI attempts to unravel an AI application’s “why” and “how” to help humans understand how the model arrives at its decision. The goal is to make humans more comfortable and trust the model’s logic to make decisions.
Explainable AI helps end users understand an AI model, the intent for which it was created, and the expected output. Since these models are the handiwork of synthesized data, it is critical to understand the implications of utilizing such models in real-world scenarios. In the case of enterprises, explainable AI will be used to verify, govern and track how AI models arrive at their decisions. It attempts to bring the black box of testing into a transparent glass box so that everyone can see, understand, and trust the steps involved in arriving at decisions. One bad move can spell huge losses, distrust, and lowered CX. Technology evolutions will demand innovative business models, and enterprises will increasingly depend on explainable AI.
2.3 Enterprises will be using MLOps to bridge the gap between machine learning and data engineering
ML (Machine Learning) is the core process that helps create models and datasets with which the model trains. The model is fed numerous datasets to help sharpen its prediction curve, accuracy, eciency, and scalability. But the problem lies in governance once these models are implemented, giving rise to a lack of ROI and reducing its scope to a costly experiment. In a real-world scenario, enterprise businesses need the help of many models and a ton of training data. The speed, accuracy, and eciency in turning these models into viable revenue are where MLOps as a practice can help. There’s also the latent need to scale ML operations to the business needs and demands.
MLOps, or Machine Learning operations, is simply a combination of machine learning and DevOps principles. It combines machine learning and data engineering practices to eectively engineer and deploy ML models. MLOps concerns itself with the entire ML lifecycle, model generation, CI/CD practices, delivery, and deployment with analytics and governance. The diagram below shows how the lifecycle of MLOps.